Latest News
Four examples of how digital transformation has impacted life science R&D
17 Apr, 20244 MinutesThe life science industry is under immense strain in the face of pandemic recovery, an aging...
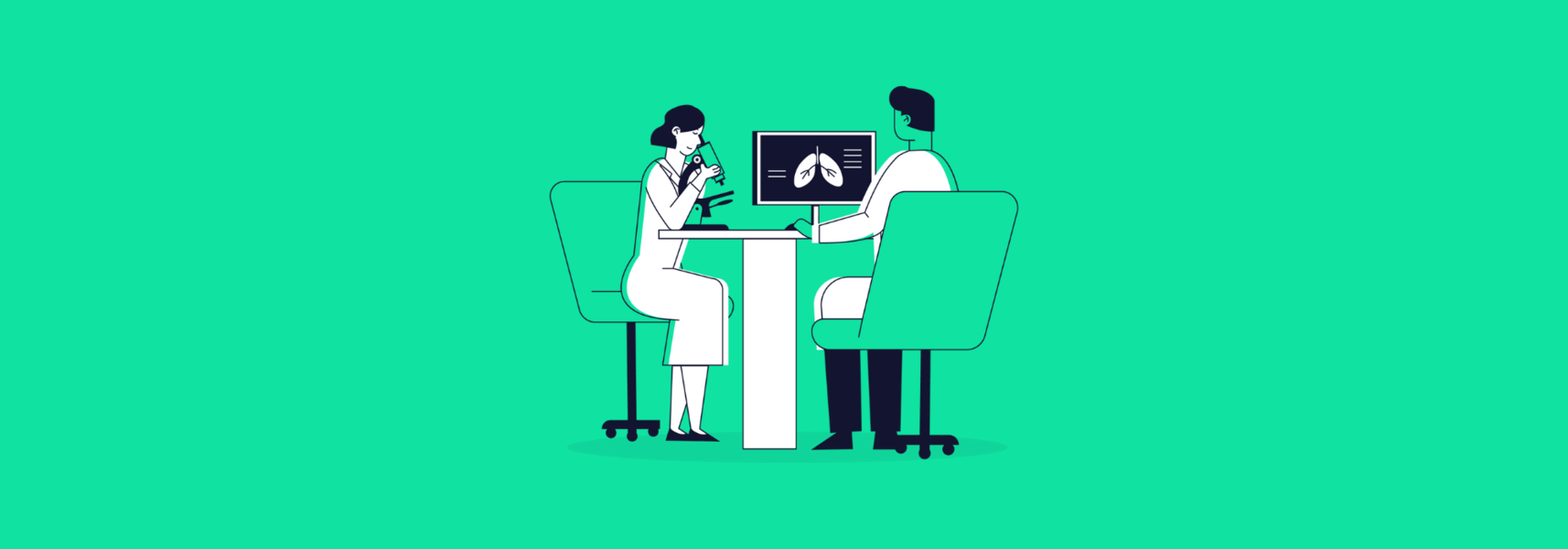
The life science industry is under immense strain in the face of pandemic recovery, an aging population, rare disease concerns and market access pressures. Digital transformation strategies are being introduced across the entire drug development cycle to respond to these challenges, and promising use of digital technologies paint the picture of a utopian future where technology, data and digital strategy combine to create a streamlined healthcare effort that addresses more patient needs within a shorter timeline.
The impact of digital transformation can be felt throughout the entire product development chain, from discovery and development through to clinical trials and reporting. These developments can influence the structure of departments or entire organisations, create new roles, and change the skillsets required for existing life science jobs.
New avenues created through digital advancements in the life science sector present an opportunity for those in the R&D space to improve processes, efficiencies and returns, and improve the experience of patients across the globe.
Here are four innovative examples that set a solid standard for the application of AI, machine learning and other digital technologies in a research and development setting.
Accelerating drug discovery using Artificial Intelligence
The drug discovery process is slow, expensive, and typically sees less than 10% of its products approved for use on the patient population. Early adoption at the discovery phase has indicated that, applied in the right way, AI can help improve product timelines and reduce the financial capital needed in the R&D stage.
In June 2023, Isilico Medicine's drug candidate for the treatment of pulmonary fibrosis, INS018_055, became the first entirely AI-discovered and AI-designed drug to enter a phase 2 trial, representing an important milestone for the industry.
The preclinical candidate was selected in February 2021, just 18 months after the project began. Nine months later Insilico announced first-in-human for phase 1 trials. It was a milestone in drug discovery – from novel target discovery to Phase 1 in under 30 months, about half the time the process would take with traditional drug discovery, thanks to the speed and efficiency provided by AI, and for a fraction of the cost.
This venture is a great example of how sophisticated AI technology alongside deep knowledge of a therapeutic area can create impressive outcomes, and such collaborations between leaders in the digital space and R&D giants will become commonplace as we delve deeper into the capabilities of AI and machine learning.
Integrating and compiling trial data using machine learning
Historically, compiling trial data to produce reports or case studies has required manual data input sourced from multiple locations. As such, inconsistencies and errors can be inputted, causing documents to be revisited or resubmitted, halting trial progress or delaying start-up processes.
Right now, one of the most common applications of AI in a life science setting involves analysing and processing such data.
Back in 2019 Pfizer organised a hackathon to test the machine learning technology of four potential partner companies, where the singular goal was to identify errors and discrepancies from the datasets of 30 clinical trials.
Pfizer disclosed to the companies the known errors in the data, so they could “teach their tools” the patterns in such errors, and used the findings from each business as an indicator for pursuing a partnership.
Machine learning technology can take data from multiple sources and use it to populate standardised forms, documents and trial artifacts that support in timely start-up of clinical trials or to further ongoing research projects.
Using deep learning to predict patient responses
There is a great amount of uncertainty when it comes to how a product will perform when prescribed to a real-world population. Deep learning workflows, however, hold promise for identifying how patients might respond based on existing data and known factors of a particular demographic.
Back in 2019 a study was conducted that set out to predict the clinical response to therapeutic agents within treatments for cancer. By using a database of 1,001 cancer cell lines, deep neural networks were trained to predict possible drug responses on multiple test groups of patient cohorts.
We can expect machine and deep learning technology to be increasingly leveraged in this way to reduce uncertainty, demonstrate early efficacy, and inform key decisions when it comes to development of new drugs.
Reimagining laboratory environments by introducing automation
Automation has a long history of reducing human input in tasks, and in turn reducing the time it takes to complete laborious assignments and reducing the capacity for human error. In an R&D setting, implementation of automation technologies can help increase productivity, accuracy, and quality compliance.
Oxford-based technology firm Arctoris combine automation with robotics to alleviate much the manual work needed to conduct experiments in a laboratory setting. Using their technology, researchers can access a platform to control their laboratory experiments remotely using robotics, which during the height of the pandemic means those that would typically be lab-based can still conduct experiments from home.
Experiments conducted this way also allows for a greater level of protocol adherence and consistency; a conventional protocol might say something along the lines of ‘mix the sample’, which would naturally be slightly different actions each time when undertaken by a human. The use of robotics in this capacity can ensure the same movement is made each time the protocol is followed.
When it comes to pharmaceutical R&D, implementing technology like wearables, AI, deep and machine learning, robotics, and automation can have a positive impact right up the value chain through to commercialisation.
Such tech promises to not only positively impact the bottom line, but bring products to patients faster, and improve the efficacy of treatments that are brought to market.